Condition monitoring and anomaly detection in cyber-physical systems
Jun 15, 2022·,,·
0 min read
William Marfo
Deepak K. Tosh
Shirley v. Moore
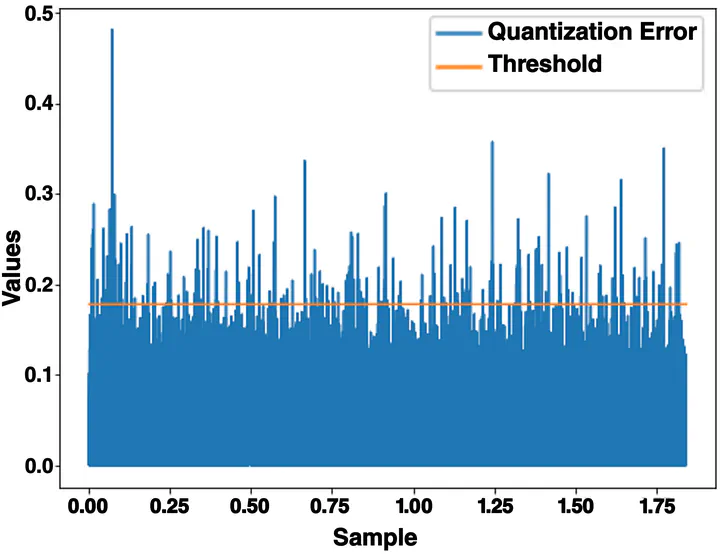
Abstract
The modern industrial environment is equipping myriads of smart manufacturing machines where the state of each device can be monitored continuously. Such monitoring can help identify possible future failures and develop a cost-effective maintenance plan. However, it is a daunting task to perform early detection with low false positives and negatives from the huge volume of collected data. This requires developing a holistic machine learning framework to address the issues in condition monitoring of high priority components and develop efficient techniques to detect anomalies that can detect and possibly localize the faulty components. This paper presents a comparative analysis of recent machine learning approaches for robust, cost-effective anomaly detection in cyber-physical systems. While detection has been extensively studied, very few researchers have analyzed the localization of the anomalies. We show that supervised learning outperforms unsupervised algorithms. For supervised cases, we achieve near-perfect accuracy of 98% (specifically for tree-based algorithms). In contrast, the best-case accuracy in the unsupervised cases was 63%—the area under the receiver operating characteristic curve (AUC) exhibits similar outcomes as an additional metric.
Type
Publication
2022 17th Annual System of Systems Engineering Conference (SOSE)