Enhancing Network Anomaly Detection Using Graph Neural Networks
Jun 13, 2024ยท,,ยท
0 min read
William Marfo
Deepak K. Tosh
Shirley v. Moore
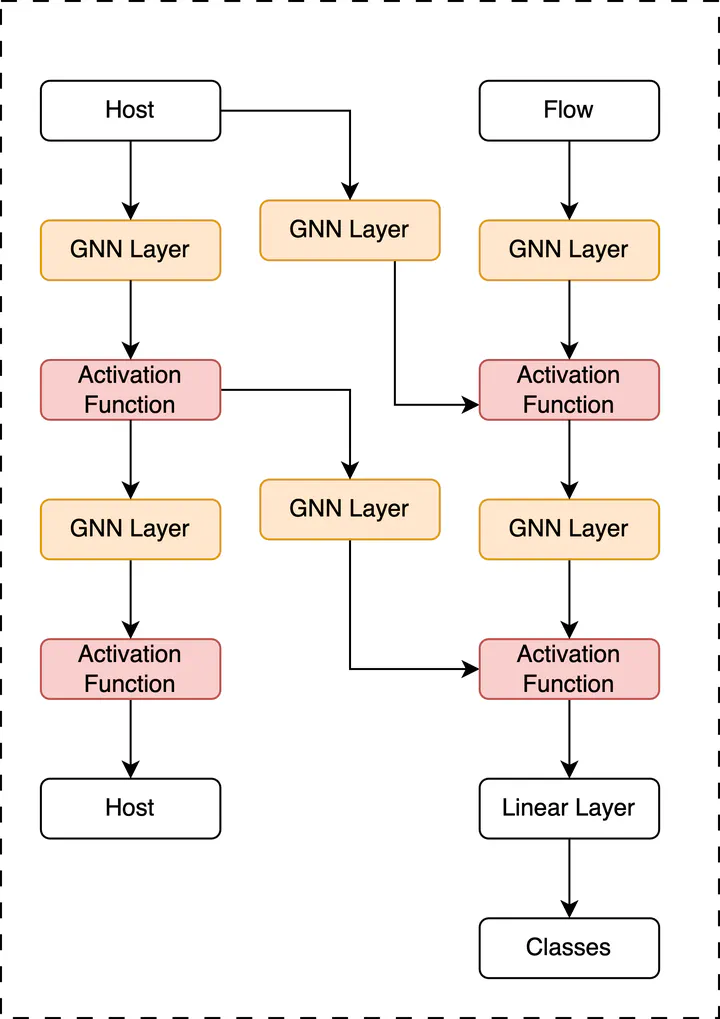
Abstract
In the world of Internet of Things (IoT) networks, where devices are constantly communicating, keeping them secure from cyber threats is critical. This paper introduces a novel approach to detecting unusual and potentially harmful activities in these networks using graph neural networks (GNNs). We combine two specific types of GNNs-GraphSAGE and graph attention networks (GAT)-to create a model that understands and represents the behaviors and interactions in a network. GraphSAGE creates an embedding of network activities by examining local data interactions, while GAT directs the model’s focus to the most critical interactions. By integrating these two methods in a single model that considers different types of interactions (both host and flow nodes), we aim to create a system that accurately represents the current state of a network and can also spot anomalies effectively while reducing false positives and negatives. Our innovative approach has demonstrated promising results, achieving an accuracy of 98% on the UNSW-NB15 dataset, significantly outperforming standalone GraphSAGE and GAT models. This underscores its potential as a robust framework for securing IoT networks against cyber threats and anomalies.
Type
Publication
22nd Mediterranean Communication and Computer Networking Conference (MedComNet)